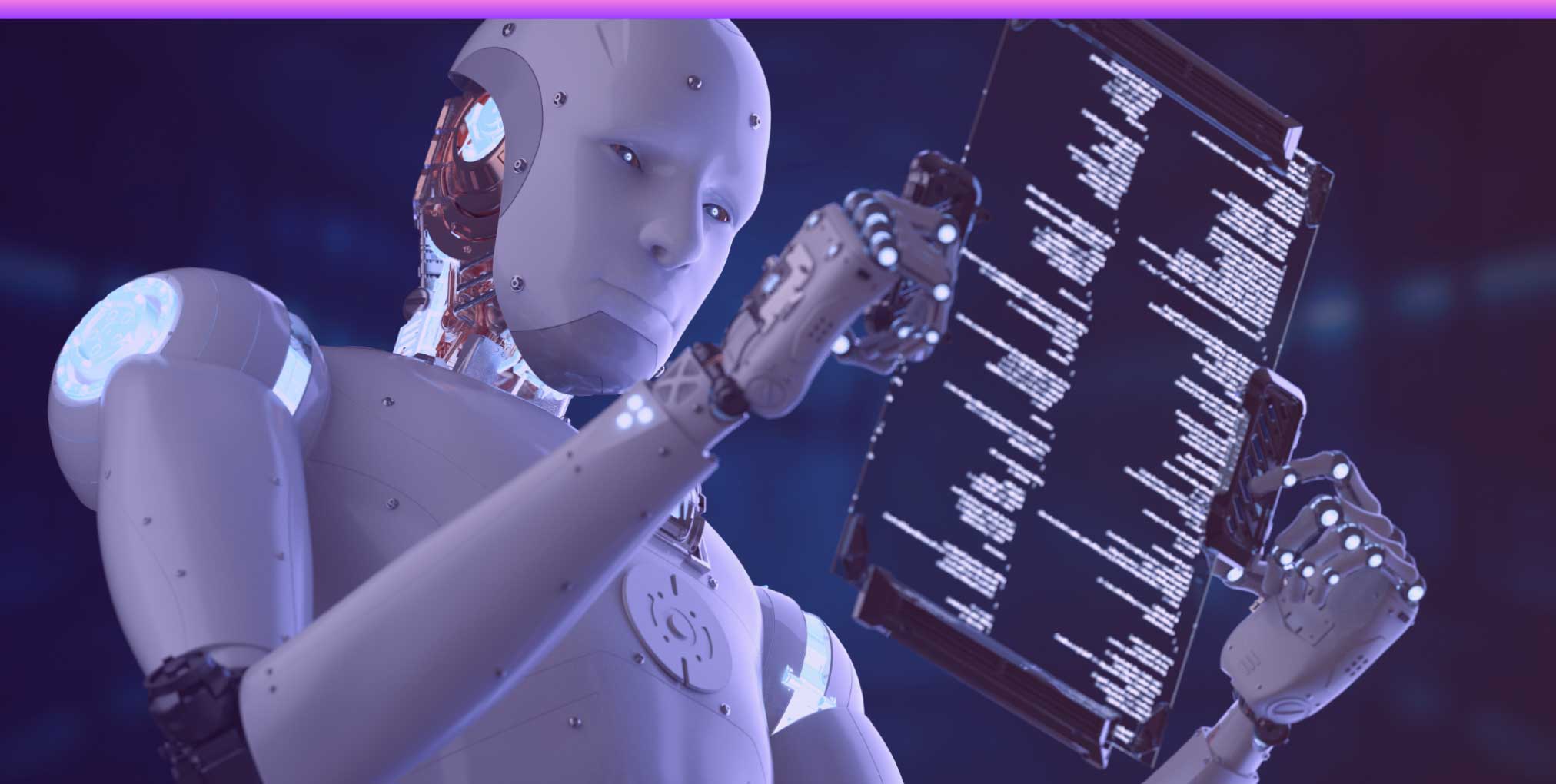
December 20, 2024
Chances are, you are familiar with generative AI already—it’s everywhere, and for good reason. But if you aren’t, you must be living in a cave trying to ignite the fire using stones. Otherwise, I am unlikely to believe you haven’t heard of this technology.
Generative AI is doing wonders for companies. It helps businesses improve productivity, be more creative, and generate more revenue. A recent McKinsey report shows that generative AI can help enterprises get 5 to 15 percent more value from the money they spend on marketing. The report also suggests that gen AI can make sales activities 3 to 5 percent more effective.
However, here’s the catch—implementing generative AI isn’t a simple process. It has its share of challenges that businesses face. And who else can help you understand generative AI challenges and their solutions better than experts with 10+ years of experience helping organizations adopt gen AI solutions?
Today, I will share the 5 most common challenges of generative AI that we face as a team when assisting organizations to adopt generative AI.
We can find the applications of generative AI across various industries—be it healthcare or marketing. However, organizations mostly face challenges in implementing generative AI. These include:
You might know that generative AI solutions depend on the quality of training data. If the data is clean, complete, and free from biases, then the outcomes will also be accurate. Without that, the predictions of the AI solution will surely be wrong.
You might have heard the phrase “Garbage in, Garbage out”—it is true in this situation. Bad data is the very challenge of generative AI that organizations fall for.
A recent survey found that AI models trained on bad data led to wrong business decisions. It caused companies to lose about 6% of their yearly earnings. For respondents earning $5.6 billion a year, this meant an average loss of $406 million.
But the question that matters to you is, “How do you overcome the data quality challenges of generative AI?”.
You can overcome data quality challenges by regularly reviewing and cleaning your data to remove any biases and inaccuracies. You can also analyze the responses of the AI model and make necessary adjustments to improve the model.
It’s impossible to talk about generative AI challenges and not mention the ethical concerns. The ethical concerns of generative AI include but are not limited to:
Keeping things short, these are some questions that arise with generative AI. How does a generative AI solution reach an output? Who will be responsible for the outputs of generative AI systems? What is the impact of generative AI on the job market?
It is important to earn the trust of society, and that’s possible by making generative AI systems explainable. You can use explainable AI techniques to understand how an AI model makes a decision. Try to create strict ethical guidelines and auditing mechanisms to make sure that generative AI is used responsibly.
Data breaches have become a normal thing these days. Do you know that 422.61 million data records were leaked in data breaches just in the third quarter of 2024?
Things become more serious when it comes to AI solutions. These systems use a large amount of data and are more vulnerable to such cyber attacks. In such a time, it is of the utmost importance to take necessary measures to prevent data breaches.
Encrypting data is a simple method that you can use to protect data privacy and security. Another way to overcome data security challenges is to create and follow clear privacy policies. You can also consider limiting the data access to authorized people. Last but not least, you can comply with international regulations like HIPAA and GDPR to protect your data.
Regulatory compliance is another challenge of generative AI that most businesses face. The reason? The rules and regulations vary for different regions and are constantly changing, making it difficult for businesses to adapt. Apart from this, intellectual property disputes may arise, leading to legal actions.
Organizations around the world are present to ensure that generative AI systems adhere to the latest rules and regulations.
To ensure regulatory and legal compliance, conduct regular audits and identify areas for improvement. On top of that, you can collaborate with experts in AI and regulations. Compliance with CCPA will also work for you.
Top Generative AI Challenges
Integrating AI and generative AI with existing systems? That’s no easy feat. One of the biggest generative AI challenges is compatibility—legacy or outdated systems don’t always play nice with modern AI solutions.
There is a high probability that your current systems will not integrate with the generative AI solution. These challenges of generative AI can slow down adoption and even disrupt operations.
To overcome this generative AI challenge, you need to plan your integration strategy ahead of time. You can use tailored APIs or middleware for smooth integration with existing systems. Consider trying your generative AI solution in a test setting first before rolling it out everywhere.
Generative AI has immense potential to help an organization pave its way toward its goals. However, to achieve this, it is crucial to get past the challenges of generative AI. In this article, I have tried to sum up the top 5 generative AI challenges that businesses often face. The best part? You already have a solution to overcome every common challenge in implementing generative AI.
Xeven Solutions is a leading AI development company that has earned global clients due to its client-centric approach. We help businesses modernize, scale, and build AI and generative AI solutions. We bring a decade of experience in helping businesses navigate generative AI implementation challenges. Our team of experts tailors gen AI solutions to meet your needs. Contact us to discuss your needs and learn how we can help you overcome the challenges of generative AI adoption.